The financial markets have always been a battleground of intellect and speed, where the slightest edge can translate into monumental profits. In 2025, this arena is witnessing the rise of two formidable contenders: quantum computing and artificial intelligence (AI). Both technologies promise to revolutionize trading strategies, risk management, and overall market efficiency. But as we navigate this technological frontier, the question arises: which of these algorithmic titans will ultimately dominate the trading landscape?
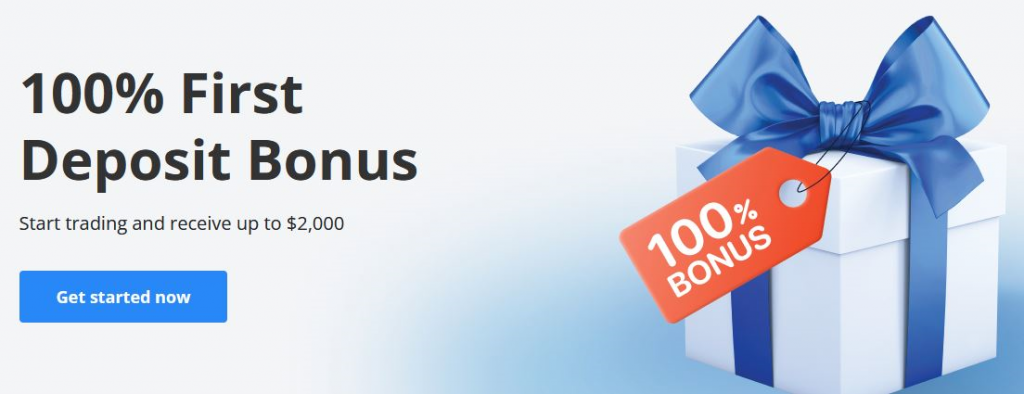
The Ascent of Artificial Intelligence in Finance
Artificial intelligence has already made significant inroads into the world of finance. Machine learning algorithms, a subset of AI, are now widely employed for tasks ranging from high-frequency trading (HFT) to fraud detection and portfolio management. These systems excel at identifying complex patterns in vast datasets, enabling them to make predictions and execute trades with remarkable speed and precision.
Current Strengths of AI in Trading:
- Pattern Recognition and Prediction: AI algorithms can analyze historical price data, trading volumes, news sentiment, and a multitude of other factors to identify subtle correlations and predict future market movements. For instance, a deep learning model can be trained on years of stock market data to forecast short-term price fluctuations with a higher degree of accuracy than traditional statistical methods.
- High-Frequency Trading (HFT): AI-powered systems are the backbone of modern HFT. Their ability to process information and execute trades in milliseconds allows them to capitalize on fleeting arbitrage opportunities and micro-price discrepancies. Imagine an AI algorithm identifying a temporary mispricing between two related assets and executing simultaneous buy and sell orders to profit from the difference, all within a fraction of a second.
- Risk Management: AI can analyze vast amounts of data to assess and manage risk more effectively. Machine learning models can identify unusual trading patterns that might indicate fraudulent activity or predict potential market volatility, allowing institutions to take proactive measures. For example, an AI system can continuously monitor a portfolio’s exposure to various risk factors and automatically adjust positions to maintain a desired risk profile.
- Algorithmic Trading Strategies: AI enables the development of sophisticated and adaptive trading strategies that can adjust to changing market conditions in real-time. Reinforcement learning algorithms, for example, can learn optimal trading policies through trial and error, continuously refining their strategies based on market feedback.
The Quantum Leap: Unleashing Unprecedented Computational Power
Quantum computing, while still in its nascent stages compared to AI, holds the potential to unlock computational capabilities far beyond the reach of even the most advanced classical computers. By leveraging the principles of quantum mechanics, such as superposition and entanglement, quantum computers can perform certain types of calculations exponentially faster than their classical counterparts.
Potential Impact of Quantum Computing on Trading:
- Enhanced Optimization Problems: Financial markets are rife with complex optimization problems, such as portfolio optimization, risk management, and algorithmic trading strategy development. Quantum algorithms, like the Quantum Approximate Optimization Algorithm (QAOA), could potentially find optimal or near-optimal solutions to these problems much faster than classical algorithms. Imagine a portfolio manager using a quantum computer to construct an investment portfolio that maximizes returns while minimizing risk across thousands of assets, a task that would be computationally intractable for classical computers.
- Faster and More Accurate Risk Modeling: Quantum computing could revolutionize risk modeling by enabling more sophisticated simulations and analyses. For instance, quantum algorithms could be used to model complex derivative pricing scenarios or to perform more accurate stress tests on financial institutions.
- Breaking Current Encryption: While not directly a trading advantage, the potential of quantum computers to break current encryption methods poses a significant security risk to financial data and communication. This necessitates the development and adoption of quantum-resistant cryptography.
- Potentially Discovering Novel Market Inefficiencies: The sheer computational power of quantum computers might enable them to identify subtle and complex patterns in market data that are currently invisible to even the most advanced AI algorithms, potentially uncovering new and profitable trading opportunities.
The 2025 Landscape: A Hybrid Approach?
In 2025, it’s unlikely that either quantum computing or AI will completely eclipse the other in the realm of trading. Instead, we are more likely to witness a synergistic relationship, where the strengths of both technologies are leveraged to create even more powerful and sophisticated trading systems.
Why a Hybrid Approach Might Prevail:
- Quantum Computing Still Developing: While making rapid progress, quantum computing is still in its early stages of development. Fault-tolerant, large-scale quantum computers are not yet widely available, and the development of quantum algorithms for specific financial applications is an ongoing process.
- AI’s Maturity and Adaptability: AI technologies, particularly machine learning, are already mature and widely adopted in finance. Their ability to learn from data and adapt to changing market conditions makes them highly versatile.
- Complementary Strengths: AI excels at pattern recognition and prediction in large datasets, while quantum computing holds the promise of solving complex optimization problems and potentially uncovering deeper market insights. Combining these capabilities could lead to breakthroughs in areas like algorithmic trading strategy optimization and risk management. For example, an AI could be used to pre-process vast amounts of market data, identifying key features and patterns, which are then fed into a quantum algorithm to optimize trading parameters or portfolio allocations.
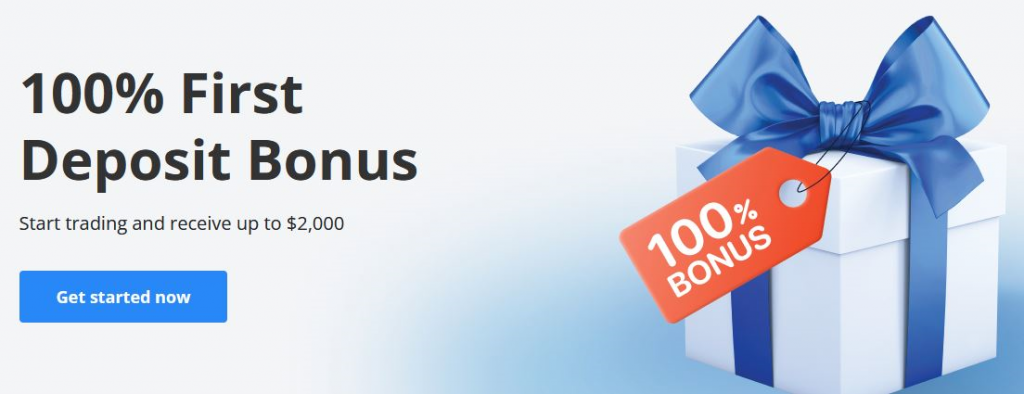
The Verdict for 2025
In 2025, artificial intelligence is likely to maintain its dominant position in the trading world. Its widespread adoption, proven effectiveness in various financial applications, and continuous advancements in areas like deep learning and reinforcement learning provide a strong foundation.
However, quantum computing will be an increasingly influential emerging force. While not yet ready for widespread deployment in high-frequency trading, its potential for solving complex optimization problems and enhancing risk modeling will attract significant research and investment. We may see early adoption of quantum-inspired algorithms running on classical computers or the use of early-stage quantum computers for specific, computationally intensive tasks within larger financial institutions.